Keynote Speakers at ICCCN 2024
July 29, 2024
Keynote Talk:
Title: Practical Integrated Sensing and Communication for 6G
Speaker: Dr. Joerg Widmer, IMDEA Networks
Abstract: The high bandwidth available with new mobile and wireless communication technologies allows for very high data rates, and at the same time enables highly accurate localization and environment sensing. This keynote highlights the practical design aspects of localization and sensing systems, and in particular the challenges of integrated sensing and communication. We discuss how to achieve decimeter-level location accuracy with simple commercial millimeter-wave off-the-shelf communication devices and how sub-6 GHz information can help to further improve the reliability of the system. We also discuss how to use communication hardware to perform zero-cost monitoring of human movement and activities in indoor spaces (rather than using dedicated radars). To this end, access points can be retrofitted to perform radar-like extraction of the tiny micro-Doppler effects caused by the human motion. Such systems can then be used to enable fine-grained sensing applications such as simultaneous activity recognition and person identification of multiple human subjects. Besides the underlying concepts, the keynote will specifically focus on the practical implementation aspects, testbed design and experimental results with such systems.
Biography:

July 30, 2024
Keynote Talk:
Title: Application Customized Networking
Speaker: Dr. Minlan Yu, Harvard
Abstract: As applications become highly distributed, the need for high-performance communication and coordination between servers becomes critical. Unfortunately, today's network abstractions and network stacks significantly limit performance optimization due to their generic for diverse applications: Traditional network abstractions focus on individual connections, missing the potential benefits of coordinating groups of connections in distributed systems. Efforts on network-layer performance optimization sometimes lead to more computation at applications. Layers of network stacks in the kernel, which are essential for security and resource sharing, introduce significant overhead.
In this talk, I advocate for application-customized networking, which empowers applications to specify their own communication needs and optimizations in the network layer. This approach enables applications to coordinate across connections, perform end-to-end optimizations, and reduce kernel overhead, all while retaining the security and resource-sharing benefits of the kernel. I will give two examples of application-customized networking: First, for distributed transaction systems, we introduce DINT, which leverages eBPF to support frequent-path transaction operations directly in the kernel. DINT achieves up to 2.6× higher throughput than using a DPDK-based kernel-bypass stack, with only a maximum of 10% increase in average unloaded latency. Second, for distributed deep learning, we introduce THC, a bidirectional tensor homomorphic compression framework that enables the direct aggregation of compressed values and thus eliminates the computational overheads of (de)compression at the parameter server. THC reaches target accuracy up to 1.47× faster compared with state-of-the-art systems.
Biography:
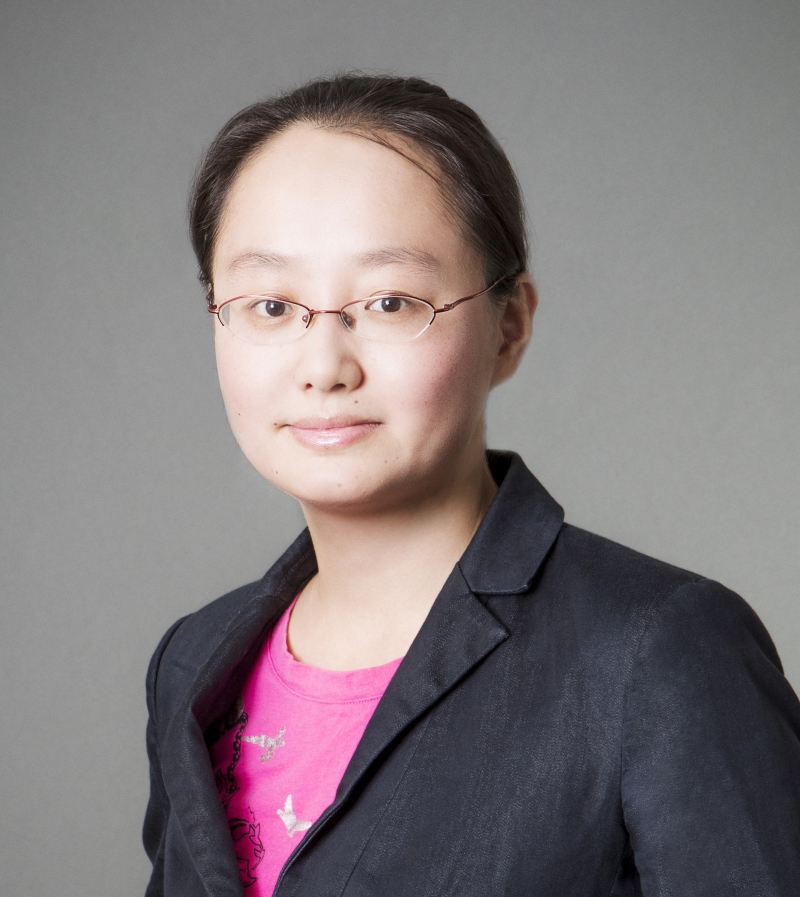
July 31, 2024
Keynote Talk:
Title: Neural Feature Learning for Engineering Problem
Speaker: Dr. Lizhong Zheng, MIT
Abstract: Using deep neural networks as elements of engineering solutions can potentially enhance the overall performance of the system. Engineering problems are often more complex than the currently popular applications of DNNs, such as image or natural language problems, in that 1) we often have domain knowledge in various forms, including constraints, preference, and loss metrics; 2) we often look for parameterized solutions for a collection of scenarios, which are all different from the one where the training data is collected; 3) we often need to process multi-modal inputs with different time scales, resolutions, or qualities; and 4) because of the tight resource constraints, we often employ more complex sample management techniques such as the use of contrastive negative samples or augmentation. Facing these fundamentally more complex situations, we argue that the existing practices that use DNNs as black boxes can be insufficient.
In this talk, we discuss a new analysis method based on an information theoretic approach to study multivariate dependence. We define a new metric to measure the semantics of the information contents carried by features learned in a DNN and, based on that, a new architecture to separate the contributions of the different variables to the multivariate dependence. We demonstrate how to use this architecture to allow domain knowledge to be incorporated in the learning process. We use the example of symbol detection in wireless fading interference channels to discuss some of the key issues and steps in such solutions.
Biography:
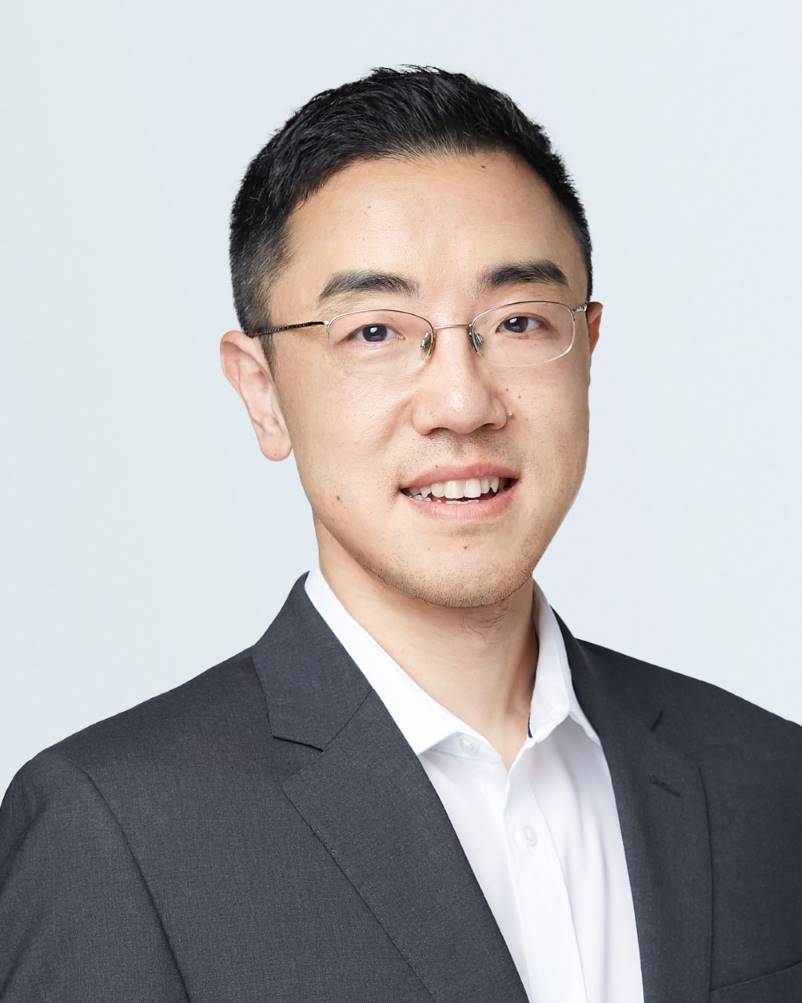